The Foundation of AI: Data at Scale “There is no AI without data, there is no AI without unstructured data, and there is no AI without unstructured data at scale,” said Chet Kapoor, CEO of DataStax, underscoring the critical role of vast and varied datasets in AI. Speaking at TechCrunch Disrupt 2024, Kapoor emphasized how scaling unstructured data is the backbone for AI to function effectively, especially in modern AI applications where structured and unstructured data intermix to fuel machine learning models. The conversation explored how companies could refine their data pipelines to support AI, featuring insights from industry experts Vanessa Larco, a partner at NEA, and George Fraser, CEO of Fivetran.
Prioritizing Product-Market Fit Over Rapid Scaling The session’s central message for companies exploring AI was clear: rather than attempting large-scale, sweeping deployments of AI, the current focus should be on targeted, incremental applications with clear, product-market fit. Kapoor noted that this restraint is essential as we’re still navigating the early days of generative AI, making large-scale AI deployments premature for most businesses. This step-by-step approach allows companies to experiment, iterate, and refine their AI applications without the burdensome risk of extensive misallocations of resources.
“Don’t be overly ambitious at first,” Kapoor advised. “Focus on practical, incremental progress. We’re still figuring it all out.” His advice reflected a practical reality: as companies are exploring how AI fits into their offerings, they must stay grounded in tangible value propositions that benefit both the organization and the customer.
A People-Centric Approach to AI Integration In discussing what truly drives generative AI’s successful deployment, Kapoor stressed that the key isn’t solely the data but the teams dedicated to building these new applications. “The most important thing for generative AI is that it all comes down to the people,” he said. These “SWAT teams” he referenced are the core players shaping the first-generation AI manuals, building expertise as they chart new pathways through trial and error.
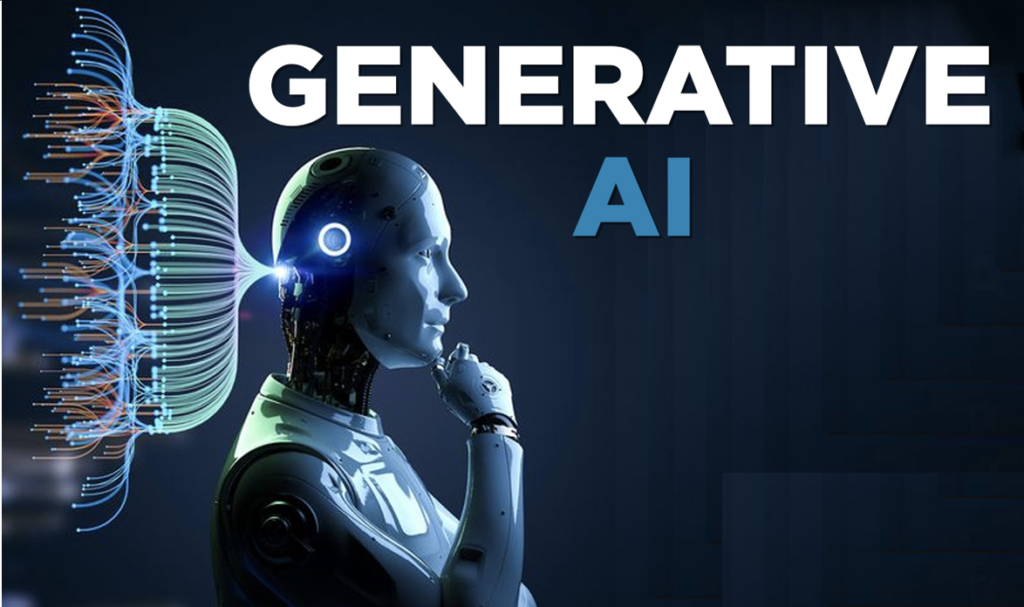
Starting Small: Pragmatic Steps for Data Utilization Vanessa Larco, who sits on boards of numerous startups, proposed a measured approach for companies, especially those new to AI. “Work backward from what you’re trying to accomplish,” Larco advised. “What are you trying to solve for, and what is the data you need?” Larco’s approach contrasts with the strategy of broad application—throwing every data set at a model in the hopes of getting a usable outcome. Instead, she recommended companies start small, focusing on highly specific internal applications that align directly with their goals. This method not only minimizes costs but also avoids the potential pitfalls of excessive or inaccurate data use.
SEE ALSO: Elon Musk’s xAI Takes Over OpenAI’s Former HQ in San Francisco
Solving Present Problems First George Fraser, who has overseen Fivetran’s growth into a data integration powerhouse serving companies like OpenAI and Salesforce, emphasized a “now” mentality in generative AI application. “Only solve the problems you have today; that’s the mantra,” he explained. “The costs in innovation are always 99% in things you built that didn’t work out.” By focusing narrowly on real issues, companies can avoid the “what ifs” and avoid wasting resources on speculative solutions. Fraser’s approach enables companies to test AI’s utility without compromising existing operations or breaking the bank on innovations that might not yet be viable.
The “Angry Birds Era” of Generative AI Kapoor framed today’s phase of generative AI as the “Angry Birds era,” likening it to the early stages of mobile app development, where applications were impactful but not yet transformative. “It’s not completely changing my life, no one’s doing my laundry yet,” Kapoor quipped. He noted that 2024 has seen many enterprises putting small, internal AI applications into production. These early adopters are learning the ropes and refining their strategies, while laying the groundwork for a more transformative future in which AI applications may alter core business operations.
What Lies Ahead: The Year of Transformation Kapoor believes 2025 will usher in a “year of transformation,” where AI applications will extend beyond pilot projects to enterprise-wide implementations that reshape companies’ trajectories. “Next year is when people will start doing apps that actually start changing the trajectory of the company that they work for,” he predicted.
Takeaways for AI-Ready Companies With AI capabilities advancing rapidly, Kapoor, Larco, and Fraser provided valuable, practical takeaways for companies ready to dip into generative AI. Focus on achievable, targeted projects with clear objectives rather than broad and potentially unfocused applications. Build small, effective teams that can innovate, learn, and adapt. Above all, use AI to solve current issues rather than planning prematurely for future scale.
As companies venture into this “Angry Birds” phase of AI, those that embrace a focused and human-centric approach to data and AI applications are most likely to emerge as leaders when the era of true transformation arrives.
Have an inspiring startup story to share? We’d love to hear it! Get your business in the spotlight by being featured in our Startups Spotlight series. Send us your story, and let’s shine a light on your journey, challenges, and triumphs. Ready to inspire the next wave of founders? Drop us an email at emmanuel@hera.marketing and let’s make it happen!